Distribution in Context: Description, Application, and Illustration
Wanna know what sampling distribution's all about? It's a fancy (and super useful) statistical tool! Think of it as a peek into the world of possibilities by examining the range of results you get from multiple samples drawn from a bigger group or population.
What's the Deal with Sampling Distributions?
A sampling distribution breaks down the different outcomes that could potentially occur for a specific statistic of a population. This knowledge is crucial for entities like governments and businesses, as it helps them make better decisions based on the data they collect. Researchers use various methods of sampling distribution, such as the sampling distribution of the mean.
How does it work?
Imagine you're a government analyst trying to figure out what infrastructure projects to invest in. You can't gather data from every citizen, so you take samples instead. That's where sampling distributions come in! They help you understand if an event or outcome is likely to happen based on factors like the sample size, sampling process, and the overall population.
So, you gather a representative sample from the population. Then, you compute a certain statistic (like the standard deviation, median, or mean) from that group. Next, you establish a frequency distribution of each sample and plot it on a graph. Once you analyze the data, you can make informed decisions about your next steps.
Special Considerations
The number of observations in the population, the number of observations in the sample, and the sampling process all impact the variability of a sampling distribution. The standard deviation of a sampling distribution is called the standard error.
Even though the mean of a sampling distribution is the same as the mean of a population, the standard error varies depending on the population's standard deviation, the size of the population, and the size of the sample. The more spread out the individual sample means are, the closer the sample mean is to the population mean.
Determining a Sampling Distribution
Let's say a researcher wants to compare the average weight of babies born in North America from 1995 to 2005 with the weight of babies born in South America during the same period. To do this, they take random samples of 100 babies born on each continent, calculate the sample mean, and repeat this process for multiple samples. They can then find the sampling distribution by mapping out the distribution of these sample means.
Types of Sampling Distributions
There are three main types of sampling distributions:
- Sampling Distribution of the Mean: This shows the distribution of means across multiple samples. It represents the average of the overall population.
- Sampling Distribution of Proportion: This involves calculating the proportion of the sample and comparing it to the proportion of the larger group.
- T-Distribution: Commonly used in cases of small sample sizes or when there's limited information about the population, the t-distribution helps make estimates about the mean and other statistical points.
The Fantastic World of Statistics
In statistics, a population is the entire pool from which a sample is drawn. It can be people, objects, events - you name it! Knowing the sampling distribution can help you make sense of those big, complicated numbers and make informed decisions. Happy analyzing! 🎉
And there you have it! Embrace the power of sampling distributions, and turn raw data into insights you can trust. 📈💡✨
- In the realm of finance, decentralized finance (Defi) enthusiasts often utilize sampling distribution concepts to examine the outcomes of various projects or tokens, promoting informed decision-making within the Defi ecosystem.
- As a Canadian, eager to further one's education and self-development, online learning platforms can offer opportunities to understand sampling distributions, enabling smarter data interpretation and more effective decision-making in personal or professional contexts.
- For a miner, understanding sampling distributions can provide valuable insight into the variability in token yields, allowing for more efficient and strategic token mining methodologies.
- In the world of education, students learning about statistics can explore how sampling distribution concepts apply in different fields, such as social sciences, economics, and more, helping them grasp statistical principles for responsible and impactful data analysis.
- A researcher may employ different sampling distribution types, such as the sampling distribution of the mean, when studying newborns' health metrics, allowing for the detection of trends, correlations, and differences between populations.
- For investors analyzing ICOs (Initial Coin Offerings), being aware of sampling distributions can help evaluate potential risks and returns by understanding the variability in offerings from multiple ICOs and making more informed investment decisions.
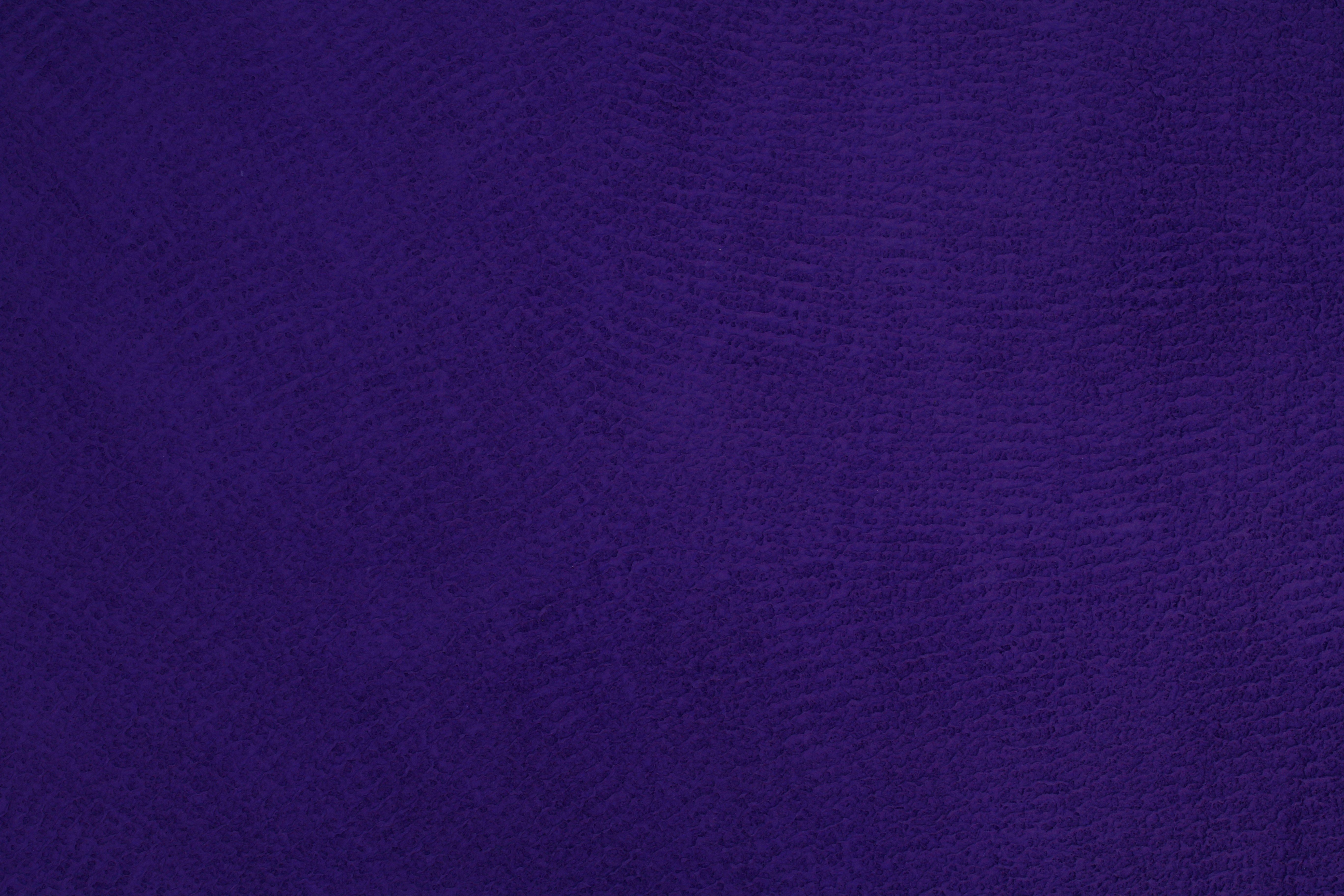